Greetings Everyone,
We are so excited to share details of our WILDLABS AWARDS project "Enhancing Pollinator Conservation through Deep Neural Network Development" and establish a place to share details along the way and gain input from this phenomenal collective in conservation tech! To start, below is a brief description of the project and our objectives. We look forward to sharing more details as they unfold!
--------
Project Description: Ocean Science Analytics (emerging from underwater environments to assist soil-side) in collaboration with The San Diego Pollinator Monitoring Program (SDPMP), led by Christina Simokat, will be developing deep neural network to detect pollinators within video data. The SDPMP conducts surveys of plant-pollinator networks in San Diego County's sage scrub communities, aiming to understand pollinator species and their interactions. Using camera traps and the deep learning technology VIAME (originally developed for underwater applications), the program collects video data on pollinator activity, particularly focusing on endangered species like Encinitas baccharis and post-fire recovery networks (check out our ArcGIS Dashboard from a recently conducted post-fire network composition study wherein we collected video data in summer 2023). This project's objectives include annotating tracks of insects in video data meticulously and developing neural networks for accurate detection of pollinator activity in large datasets, aiming to advance understanding of insect-plant interactions and potentially influencing policy changes and scientific advancements in pollinator conservation. Success entails optimizing technology for wider use, comprehensively evaluating pollinator-plant interactions, and uncovering insights into pollinator resilience and adaptation to environmental disturbances.
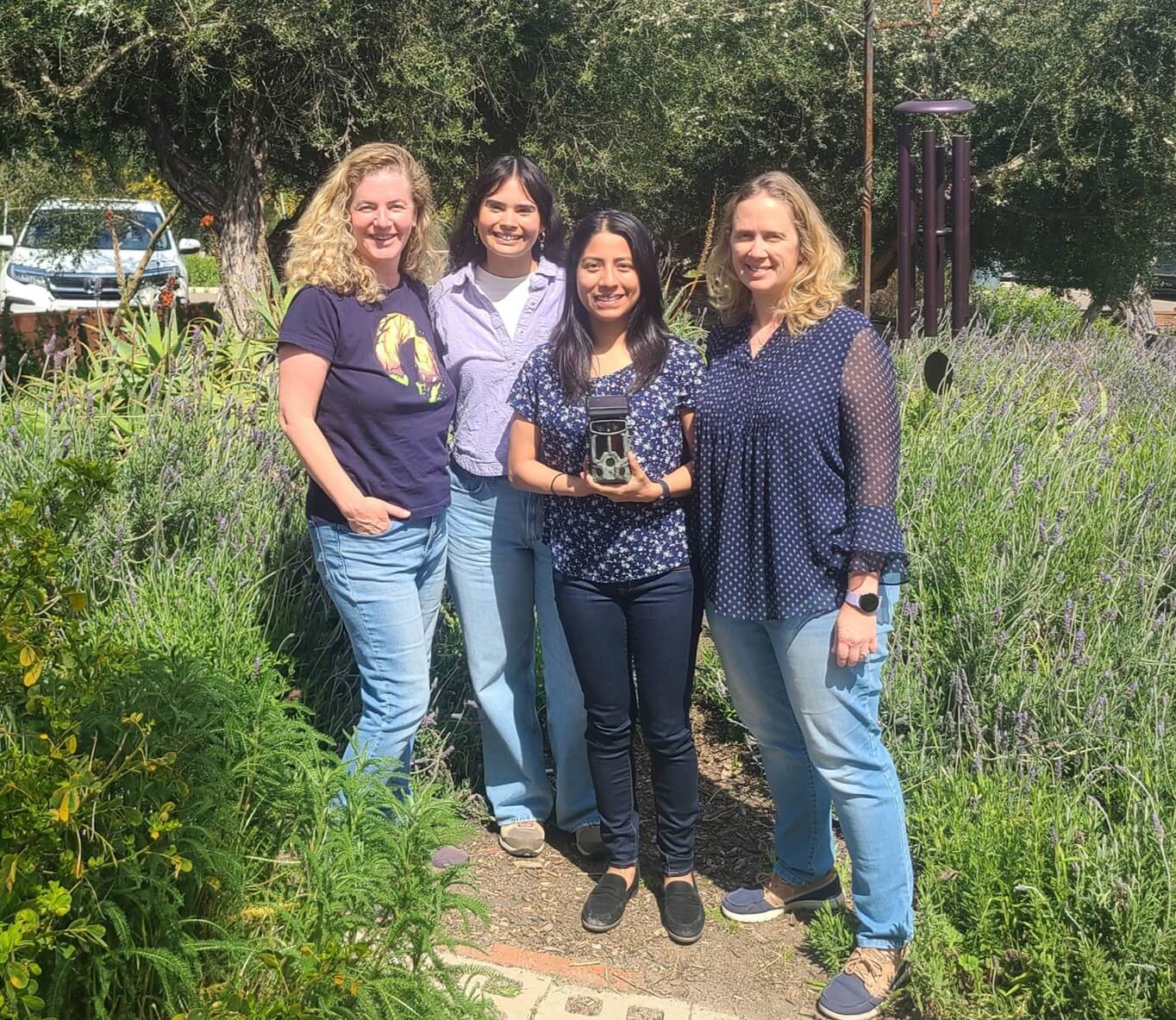
Our WILDLABS AWARD Team (from left to right): Christina Simokat, Mia Lorence (@mia_lorence), Yuli Martinez (@martiyu), and Liz Ferguson
23 November 2024 5:38pm
UPDATE: After many painstaking hours of pollinator annotation by Yuli Martinez (@martiyu ) and Mia Lorence (@mia_lorence ), and guidance from the folks at Kitware who developed VIAME, we are starting to see some promising pollinator networks! Stay tuned for our project findings in the near future - we can't wait to share!

Liz Ferguson
Ocean Science Analytics
17 April 2025 5:57pm
The Results Are In....
Hi WILDLABS Community,
We’re excited to share the results of our WILDLABS Awards project, Enhancing Pollinator Conservation Through Deep Neural Network Development — where we explored how AI and conservation technology can bridge ecosystems, applying tools originally designed for the ocean to challenges on land.
At Ocean Science Analytics, we decided to test out deep learning methods for the first time in a terrestrial context, using Kitware Inc.’s VIAME to track insect pollinators—critical yet declining contributors to biodiversity and agriculture—at the San Diego Botanic Garden.
🐝 What we did:
- Annotated insect-pollinator videos focused on the endangered Baccharis vanessae
- Trained and tested neural networks using VIAME
- Evaluated performance across day/night videos to identify conditions for improved detection
- Developed a cloud-based annotation workflow using Kitware’s DIVE platform hosted on AWS
🔍 Key takeaways:
- Nighttime models significantly outperformed daytime ones, likely due to improved contrast
- Even with limited training data, our networks showed promise in tracking insect movement
- A structured human-in-the-loop annotation process remains essential for accuracy
- This project demonstrates how existing conservation tools can be creatively adapted to new applications
This effort has laid the groundwork for scalable, AI-assisted monitoring of pollinators and revealed valuable lessons for others working at the intersection of ecology and technology. As a next step, we aim to refine these models further and support other groups in applying deep learning to insect tracking and biodiversity monitoring.
🌼 Learn more and read the full report here:
Pollinator Studies
We’re incredibly grateful to WILDLABS and Arm for funding this project and to Kitware for their guidance and support. We’re excited to continue pushing the boundaries of conservation technology across environments—land, sea, and everything in between.
Thanks for reading and please reach out via the discussion board with questions, etc., as we would love to hear from you!
— Liz Ferguson and The OSA/SDPMP Team
@martiyu @mia_lorence
Liz Ferguson
Ocean Science Analytics