Consider marine wildlife monitoring in coastal waters near California. Daily video footage analyzed by a standard ML model shows 2 octopuses, 1 shark, and 10 jellyfish - broad taxonomic categories. But species-level identification could reveal crucial details. Is that an Octopus rubescens, commonly found in this area, or is it an Octopus cyanea usually only observed in warm, tropical waters near Hawai’i? Accurate species classification is essential for understanding ocean ecosystems.
Hierarchical classification—architectures that structure data to capture relationships across taxonomic ranks (e.g., from broad categories like families to specific species)—can significantly improve classification accuracy, as demonstrated by recent advances in machine learning. In the field of marine ecology, accurate taxonomic classification is essential for addressing fundamental questions: What species exist in a particular place? What is the ecosystem biodiversity and how does it change over time?
FathomNet is hosting a Kaggle competition to find new innovative solutions to this challenge!
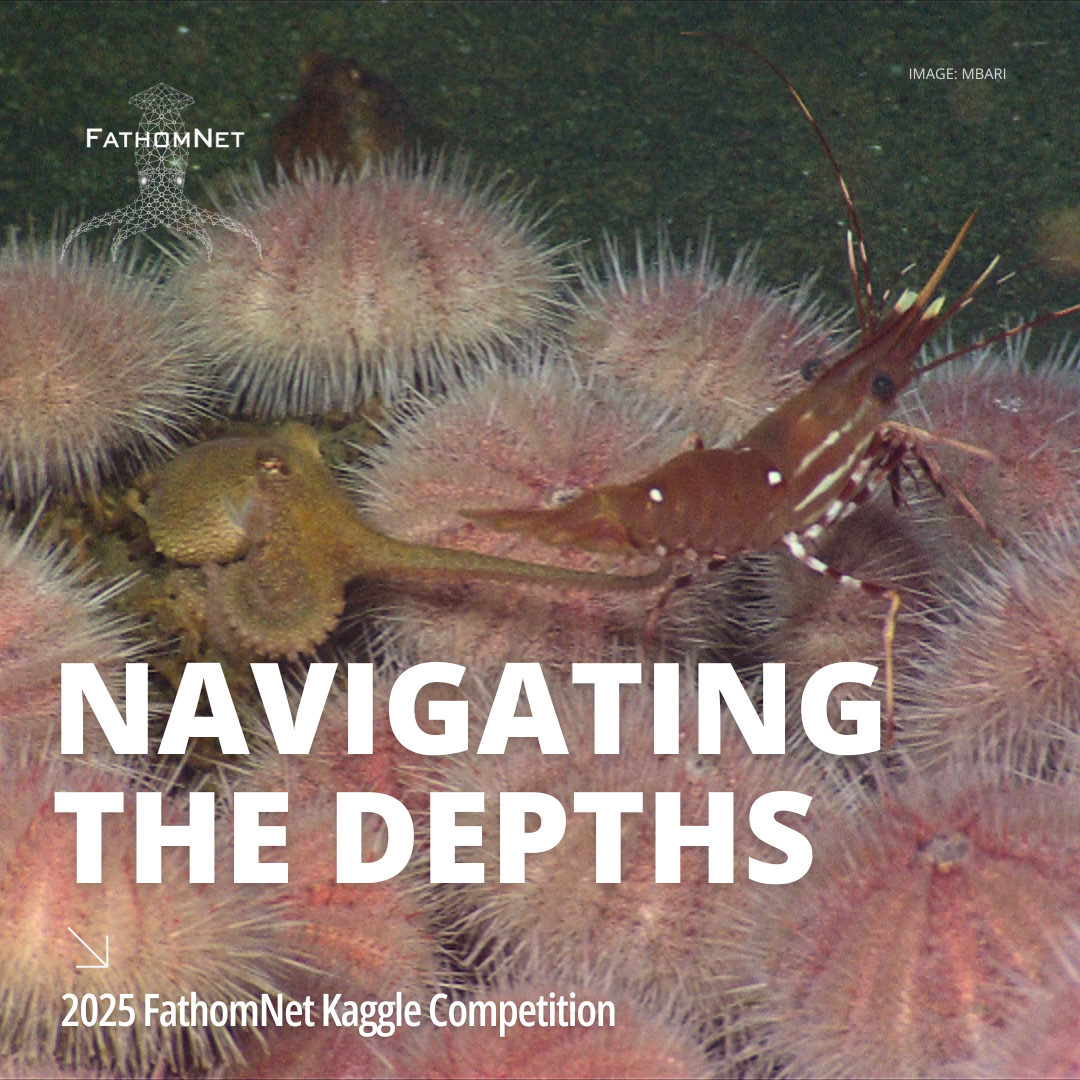
For this competition, we have curated data from the broader FathomNet image set to tackle hierarchical classification. The training set contains 79 categories of marine animals of varying taxonomic ranks (e.g., family, genus, species), wherein 300 example instances of each category are provided for training. The test set contains the same 79 categories, where roughly 10 example instances of each category are provided for evaluation. The challenge is to develop a model that can accurately classify these taxa and ideally leverage their taxonomic information to do so. Developing these solutions for ocean research will enable scientists to process and explore ocean data more efficiently.
Are you ready for a challenge? Would you be interested in forming a team to join the competition?
(Image credit: MBARI)
4 March 2025 10:13pm
Lilli Wakinekona Carlsen